When Machines Learn Hate: The Dark Side of AI and Algorithmic Bias
This article explores the phenomenon of algorithmic bias in artificial intelligence, examining how biases embedded in AI algorithms can lead to discrimination and societal harm. It highlights case studies where AI has failed due to these biases, discusses the ethical implications of biased AI, and presents strategies for creating fairer, more inclusive AI systems. The piece serves as an urgent call to action for developers, policymakers, and society to address and mitigate the risks of algorithmic bias, ensuring AI technologies advance equity rather than perpetuate injustice.
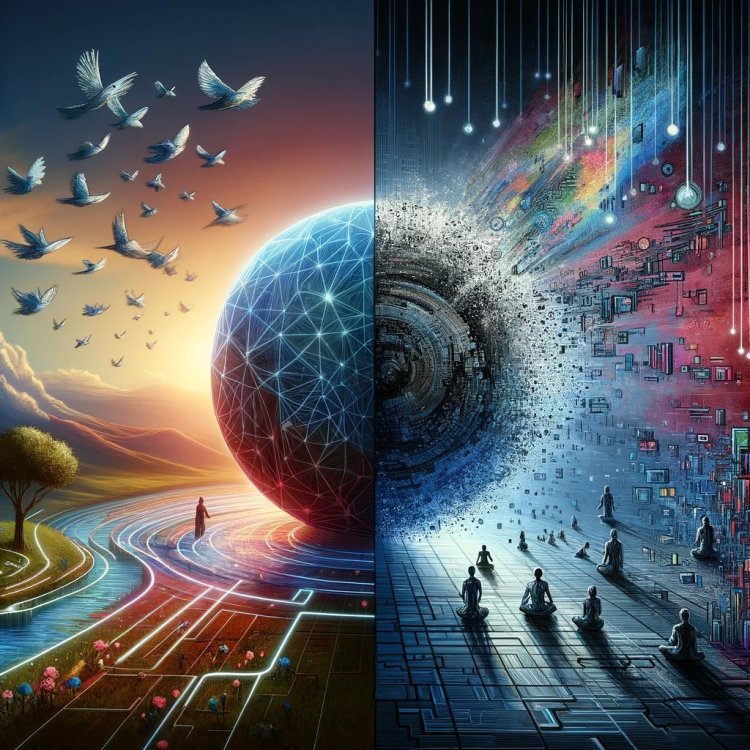
As artificial intelligence (AI) continues to intertwine with the fabric of society, its capacity to shape our world grows exponentially. Yet, within this technological marvel lies a stark warning: the danger of algorithmic bias. This article, "When Machines Learn Hate: The Dark Side of AI and Algorithmic Bias," delves into the shadowy recesses of AI development, where biases embedded in algorithms can perpetuate discrimination and inequality.
The Genesis of Bias in AI
Algorithmic bias occurs when AI systems, reflecting the prejudices present in their training data or their creators' unconscious biases, make unfair decisions. From hiring practices to loan approvals, and law enforcement, the repercussions of biased algorithms are far-reaching, affecting lives and perpetuating systemic injustices.
Case Studies of Bias in Action
This article highlights several high-profile cases where AI has gone awry, from facial recognition systems misidentifying individuals of certain ethnic groups to recruitment tools favoring male candidates. These instances serve as cautionary tales, illustrating the real-world impact of algorithmic bias.
The Ethical Imperative for Fair AI
The fight against algorithmic bias is not just technical—it's profoundly ethical. It challenges developers, policymakers, and society to reflect on the values we wish to uphold in the digital age. This section explores the ethical frameworks and governance models proposed to guide the equitable development of AI technologies.
Bridging the Gap: Solutions and Strategies
Addressing algorithmic bias requires a multifaceted approach, involving more diverse data sets, transparency in AI operations, and the inclusion of diverse perspectives in AI development teams. The article outlines innovative solutions being implemented around the globe, from algorithmic audits to community-driven AI initiatives, showcasing efforts to mitigate bias and promote fairness.
A Call to Action
"When Machines Learn Hate: The Dark Side of AI and Algorithmic Bias" serves as a clarion call to recognize and address the systemic issues within AI development. By fostering an environment of awareness, accountability, and action, we can steer the course of AI towards a future where technology amplifies justice rather than inequality.
This critical exploration is accompanied by a thought-provoking image that contrasts the potential utopia AI could offer against the dystopian reality of its misuse. It serves as a visual metaphor for the dual paths that lie before us: one towards equitable advancement and the other towards continued social injustices.
What's Your Reaction?
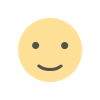
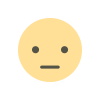
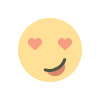
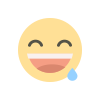
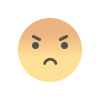
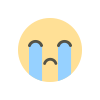
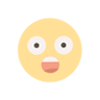